ERC grantee Martin Vechev, originally from Bulgaria, is a computer science professor at ETH Zurich and the leader of its research lab working in the field of artificial intelligence (AI). His ERC-funded project was the first to combine advanced programming languages with machine learning technics, aiming to fundamentally change how developers build software. Based on the results of his ERC project, he co-founded a start-up that was recently acquired by a leading cybersecurity company. In this interview, Vechev talks about his ERC-funded work, AI breakthroughs, and Europe’s future in the field of AI.
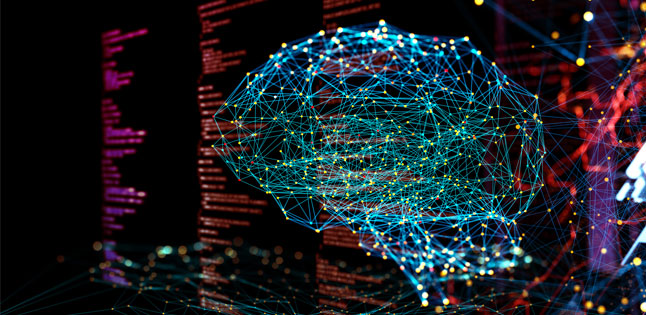
Your ERC-funded BIGCODE project aimed to change the way developers build and reason about software. Could you tell us more about it? With the project nearly finished, did you achieve what you set out to do?
My team and I combine two rather different computer science worlds, advanced programming languages and machine learning, to develop new probabilistic models for learning-based systems. These are the systems able to automatically learn and improve from their experience.
Our project built numerous such systems and was instrumental in shaping and creating a new sub-area of learning, triggering follow-up research in the process. Some of the systems we built during the project are already widely used. For example, JSNice for converting a complicated code into a simple form has hundreds of thousands of users.
Based on the results of the BIGCODE project you co-founded a spin-off called DeepCode, which was recently acquired by a billion-dollar company in cybersecurity. What does DeepCode do?
The success of the big code systems we built in academia encouraged us to create an even more broadly applicable tool. One from which every developer and company in the world could benefit. We wanted to address the issue of programmers spending a lot of time on reviewing and fixing code, rather than creating new one. This is how DeepCode came to life in 2016.
To explain what DeepCode does, we could use an analogy with Grammarly, a system that takes as input a sentence (code in our case) and produces automated suggestions for how to fix the sentence (the code). Our technology automatically examines billions of lines of code found in open source repositories and learns how to detect and repair defects in new, unseen, code. This is unlike any other system today.
What makes your work frontier research?
I think our project successfully shows what ERC grants are all about. We developed fundamentally new techniques that helped expand a new area in science. Our project built widely used systems demonstrating the potential of the methods, and the international scientific community recognised our work and breakthroughs at the highest level. This project ultimately helped create a successful high-tech start-up, leading to more jobs and opportunities in Europe.
We also received various awards for our frontier work. The one I am most proud of is the prestigious ACM Doctoral Dissertation Award given to my former PhD student Veselin Raychev. It was only the third time in the 40-year history of the award that a European PhD was recognised.
Your project's long-term goal is building a system that could write sophisticated code by itself, which would be a step towards general AI. How far are you from achieving this goal?
We are still decades away from achieving artificial general intelligence (AGI) capable of understanding and performing any task that a human can. By ‘we’, I mean any research label or a company, not just my lab. It is because the breakthroughs behind AI technology in the last few years were happening in closed world domains, where the AI models were trained and optimised for a specific task.
For example, OpenAI Five won against the best human team in the Dota 2 (an online battle video game) in 2019. It was in a setting where the game was fixed and rules were known. The same applies to programming: so far, AI can only perform tasks where the programming language and the task are fixed a priori.
While we can build AI models that solve specific tasks well, even better than humans do, this is typically possible because AI learns from massive datasets by finding useful patterns and shortcuts, not because it possesses cognitive abilities to generalise from its prior knowledge.
Many AI experts claim that the development of GPT-3 – a language model that uses deep learning to produce human-like text – is last year's biggest AI breakthrough. Would you say that your current approach to general AI is still competitive?
Yes, not only is our approach competitive, it is significantly superior to what GPT-3 can achieve and will be able to achieve with its current approach. For example, models like GPT-3 completely fail when tasks require abstraction and reasoning. While this system might be able to provide statistically likely solutions, it cannot reason about them.
In our work, we focus on building the next generation AI systems. We combine the strength of traditional symbolic search techniques that are an effective approach for developing AI system’s reasoning capabilities, and the more recent statistical methods that allow systems to learn from data.
How do you see the impact of AI on labour markets?
AI could enable humans to focus on what they are good at – being creative – while letting machines do the repetitive work that can be automated. I also expect advancements in AI to create entirely new jobs. For example, those involving deeper interactions with AI agents to accomplish a task.
What could be the biggest strength in the European AI industry's future, and what still needs to be improved?
Europe could establish itself as a leader in certain areas of AI where it is traditionally strong and which are becoming more and more important. These are, for example, safety, robustness and fairness of AI models. However, what could perhaps still be improved is the AI start-up ecosystem, where experimenting with new AI products is still more easily done in the U.S. I believe this is changing rapidly, with the ERC being a very important ingredient for closing the gap.
How important was the ERC grant for the success of your research?
The ERC grant enabled us to focus on the core research questions over a longer period, contributing to a conceptual and fundamental level of our research, but also to our ability to build systems from beginning to end. This kind of freedom was instrumental to achieving our goals and establishing Europe as a leader in this field. As I have spoken before at the European Parliament on this topic, I believe the ERC is a key ingredient for helping drive deep technological innovations.